Introduction
As financial fraud continues to rise in sophistication, institutions need more advanced analytics to keep pace. This article explores how combining rule-based systems, machine learning, and big data capabilities can enhance fraud prevention in a dynamic threat landscape.
Fraud detection is a critical aspect of maintaining the integrity and security of financial transactions and systems. A combination of rule engine, machine learning, and big data can significantly enhance fraud detection capabilities by leveraging the strengths of each approach.
Rule Engine
Traditional rule-based fraud detection relies on predefined thresholds and criteria defined by domain knowledge experts. While these static systems struggle to adapt as fraud evolves, they also face difficulties managing large volumes of data.
Machine Learning
Machine learning provides predictive capabilities by identifying complex patterns in data. But model accuracy depends heavily on large and representative training data which can be scarce. Models also lack interpretability.
Big Data
Big data architectures like Hadoop and Spark enable storing and processing huge datasets at scale. This allows machine learning algorithms to train on rich data for better fraud pattern recognition. Pipeline and streaming tools facilitate model deployment on real-time flows.
Data Analytics
Obtaining insights based on data is one of the main advantages of using big data analytics. These revelations make it possible to spot trends and patterns linked to fraudulent activity from a large chunk of data of varied sources and formats.
A Powerful Combination
Together with Machine learning, domain engines (rule engine) and big data technologies developing detection models adapted to evolving fraud trends unique to the financial institution and geography comes easy.
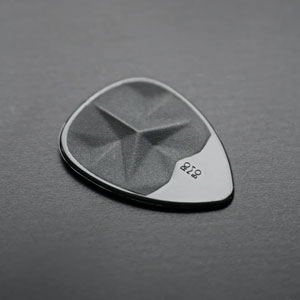
Feature One
Business rules to codify logic for simple known fraud types
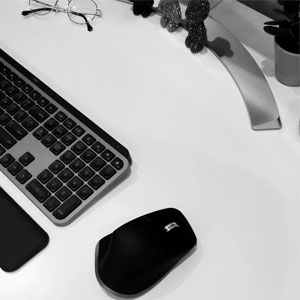
Feature Two
Big data pipeline for streaming transactions to machine learning models
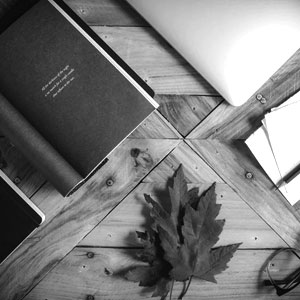
Feature Three
Scalable cloud infrastructure for storage and distributed model training
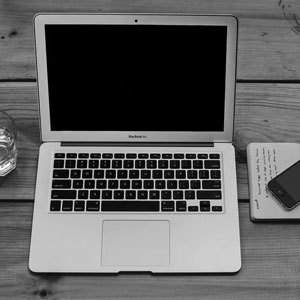
Feature Four
Machine learning algorithms to detect novel fraud behaviors and enhance accuracy over time
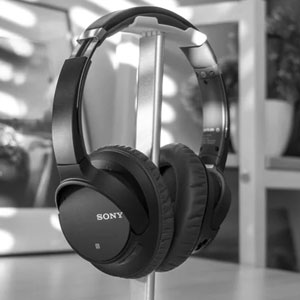
Feature Five
Ongoing model improvement loops leveraging updated data
Benefits of this unified approach include greater model accuracy, faster model retraining, reduced computational expenses, and the ability to incorporate human domain expertise.
The Future of Fraud Detection
Fraud will continue to increase as digital adoption increases. As fraudsters continue innovating, so too must fraud systems and financial enterprises. Integrating rule-based knowledge, machine intelligence, and big data capabilities alongside data analytics provides a multilayered approached to defense tailored to local needs. This empowers institutions to manage fraud risks better while providing customers with transformative products and security. The future looks brighter for financial inclusion and integrity through responsible AI application.